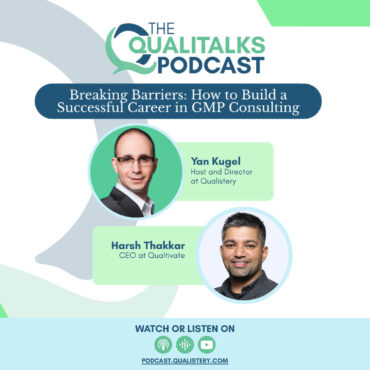
Breaking Barriers: How to Build a Successful Career in GMP Consulting [Harsh Thakkar]
Our guest Harsh Thakkar shares valuable insights on building leverage, creating content, and the importance of online presence in the business world.
Yan Kugel is joined by Fabrizio Maniglio, an industry Business Development Director at Honeywell with a wealth of experience in Quality and Supply Chain Management within the life sciences sector. Here Fabrizio discusses his journey from working in quality systems to industry and business development, and his current role in influencing and driving change within the industry. He talks about the importance of connecting systems and data in meaningful ways, and treating AI models like employees, with onboarding and downstream controls. He emphasizes the need to have an outcome in mind when using AI, and shares sources for keeping up with AI trends. The conversation concludes with the idea that life sciences companies are embracing cutting edge technology to improve outcomes for patients.
Table of Contents
Fabrizio and Yan delved into the potential impact of AI in the pharmaceutical industry, touching on its use in research, risk management, and predictive capabilities. Fabrizio emphasized the importance of understanding the intended use of AI and the need for downstream controls to catch any mistakes.
During the conversation, Fabrizio highlighted the potential use of AI in quality management, specifically in the areas of predictive maintenance and generating insights. He emphasized the need for connected data and the emergence of new insights through AI.
Fabrizio shared his insights on the future of AI in GMP and pharma, focusing on the next stages of 3 years, 5 years, and beyond. He discussed the potential for technology to be deployed to solve previously unsolvable problems and the eventual interface into data that is conversational, allowing for seamless interaction and guidance.
The conversation with Fabrizio Maniglio shed light on the transformative impact of AI in the pharmaceutical industry and the potential for AI to revolutionize quality management practices. As the industry continues to evolve, the integration of AI and the focus on outcomes will play a crucial role in driving advancements and improving overall efficiency and effectiveness.
In conclusion, the future of AI in GMP and pharma holds great potential for transforming the industry. With the right approach and a focus on outcomes, AI has the power to revolutionize quality management and drive significant advancements in the pharmaceutical sector.
Episode Chapters:
Please be advised that this is an AI generated transcript and may contain errors.
00:00 – 00:39
Yan Kugel: Welcome to our podcast episode focusing on the future of AI in GMP and pharma. Today we have a distinguished guest joining us, Fabrizio Maniglio, an experienced QA leader with a passion for IT systems and a strong background in quality and supply chain management within the life sciences sector. Currently serving as the industry business development director at Honeywell, Fabrizio brings over 15 years of hands-on experience to the table. His expertise and insights into the transformative impact of AI in the health care and pharmaceutical industries, promise to make these episodes both enlightening and engaging. Fabrizio, welcome to the
00:39 – 00:44
Yan Kugel: podcast. I hope you’re well and excited to be here.
00:44 – 00:45
Fabrizio Maniglio: Thanks for having me, Yan.
00:46 – 01:39
Yan Kugel: Great. So we did so many things already together. We ran webinars and you are a great speaker. Your webinars are amazing, informative and highly engaging. So I recommend to anybody to not miss a podcast by you and really happy to have you on the show where we can chat one-on-one. And I was curious to hear about your career. So you worked many years in the pharmaceutical sector in the quality and then you shifted to a role of a business developer at Honeywell which is a EQMS system And it’s very interesting to hear how this career shift
01:39 – 01:42
Yan Kugel: happened to you and how your role in QA helped you with this.
01:43 – 02:25
Fabrizio Maniglio: Yeah. So, if I start at the very beginning, I’m a microbiologist by trade. So I studied microbiology and then I started off my career in a lab setting. I started working in a quality control lab doing method validation. And then from there, rather quickly moved on to the dark side of quality, if you like, and joined Quality Assurance. And within Quality Assurance, I did quite a lot of different roles. I was a deviation writer at first in a deviation management team. I then took over the team. I worked in quality systems for a while and all
02:25 – 03:06
Fabrizio Maniglio: the while when I was working in quality I always had this this flair and this draw for technology and for software and for how that can be leveraged to make our lives easier at that time using the tools that I had at my disposal. Now, from there, I then went to manage a site for a while. I used to do audits and inspections as well, running back and front rooms, and also there always trying to find new solutions and technological solutions to problems. And About 7 years ago, I was involved, or 8 years ago still, in
03:06 – 03:46
Fabrizio Maniglio: the life sciences around computer systems validation. I was doing data integrity back then, just came up with a lot of different regulations. So I started doing data integrity and data and how to best ensure that the systems are maintaining data integrity. And from there, I just got the opportunity to join a quality management organization that not only was helping 1 organization, but I had the opportunity to then help many organizations solve problems through technology. So that’s what I started doing. And initially I didn’t directly start in industry and business development. I was a solutions engineer for
03:46 – 04:18
Fabrizio Maniglio: a while. And solutions engineering means taking the solutions that I had and understanding the problems and bringing the 2 together to solve problems and to deliver better outcomes. So that’s what I did for about 5 years. And then About 1 and a half, 2 years ago, I joined the industry and business development team. And now I’m in this privileged position where I can dream a little bit more about the future and what the future might bring and where the industry should be going and help influence and drive change within the industry. I hope that covers a
04:18 – 04:20
Fabrizio Maniglio: little bit all of the bases.
04:20 – 05:07
Yan Kugel: A bit. I think there are a lot of background there that 1 can discuss. So it’s very interesting that… So 1 of the webinars as well as we did together was about digitalization and you gave a lot of example, you know, of dreaming on what the future can be for the natural sciences. So, can you give some examples of what in your role as a business development director, right? So you probably also have contact with a lot of companies that you serve or different partners who you can work together because there are a lot of synergies.
05:08 – 05:22
Yan Kugel: And can you give example to some of such dreams that have been realized or you think they will be in the future and how they impact the industry, for example?
05:23 – 06:07
Fabrizio Maniglio: Yeah, so 1 thing I should preface everything with that I forgot, yes, I’m an industry and business development director at Honeywell. However, today I’m here as Fabrizio Maniglio, so anything I say in this conversation represents my opinions only and not necessarily represents or aligns with the opinions of Honeywell. But So a lot of the things that I see are trying to solve problems with efficiency and effectiveness at the very early stages when we talk to customers. But more than that, what I try to do is push the industry forward of thinking about the new problems that
06:07 – 06:44
Fabrizio Maniglio: we can start tackling, thinking about how technology has changed the way that we can potentially approach problems, and also to move into a place where maybe we go back and think, why are we doing the things the way that we’re doing them? What is the outcome that we’re looking for and find better ways of solving those problems? So that’s The majority of the discussions that I’m having with customers and with industry colleagues of mine is really around the notion of what outcomes are we looking for? Why are we doing things a certain way? And are there
06:44 – 06:51
Fabrizio Maniglio: better ways or different ways or now new ways to solve problems or maybe solve problems that were not solvable before.
06:53 – 07:39
Yan Kugel: Right. And maybe we can also separate the discussion maybe between what is and what will be. So we all seen a lot of advancement in digitalization in the pharma sector. And now we have this AI boom, especially with the large language models that are just booming everywhere. And a lot of, there are a lot of discussion on using it in the pharma sector. But then there are a lot of regulations that are being discussed now in terms of data security and data privacy and so on, and maybe other tools as well. So if we are talking
07:39 – 07:56
Yan Kugel: about what is, what can you tell us about the industry now and how it developed in the last several years and what solutions are there that are, I don’t know if we can say it’s mind-blowing, but they have completely changed the way we are doing things.
07:59 – 08:31
Fabrizio Maniglio: I think the solutions that will completely change the way that we’re doing things are yet to come. There are, especially in our industry, and you hinted a little bit about that, that though there are all these amazing advancements with the technology that we have at our disposal, I think that our industry has never really been the industry that jumps at the opportunity to be first. They always sit back and wait and see how things are developing a little bit and figure out how can we use these in a compliant way. But to answer your question around
08:31 – 09:05
Fabrizio Maniglio: the status quo, I think when it comes to digitalization, you’d be surprised to see how many companies still are not fully digital. So there’s still a lot of manual and paper processes. That’s definitely something that I was surprised with when I started having more conversations with a variety of different businesses, that there’s still a lot of manual processes. And even if you go beyond having manual processes, there’s still a lot of silos and not connected data. And I think that these are the things that we’re starting to see is the notion that 1 system can do
09:05 – 09:39
Fabrizio Maniglio: it all, I think, has gone out the window a little bit. And the acceptance, especially in recent conversations I had, was, well, we will always have multiple systems doing multiple things. But now what is going to happen is we’ll start trying to connect those systems in meaningful ways to do more with the data that is generated, or even bring more of the processes in a digital nature online so that they can be connected. Because that’s ultimately the first step, is being able to have data in a digital way. Then the second step would be to connect
09:39 – 10:10
Fabrizio Maniglio: the data in a meaningful way so that you can connect and start to understand. And there is an Autech graphic that I often reference where you have these different steps that you go through in your digitalization journey where after connecting, your journey starts and you start seeing what is happening because you have all those data points. And when you see what’s happening, you can then connect it to, well, I understand why things are happening because I see them, I know the data behind it. And understanding then leads to causality. So, you can understand why things are
10:10 – 10:38
Fabrizio Maniglio: happening. The next step is, well, if I know why things are happening, I can see where things are going. Now I can start predicting. And ultimately after prediction, if you can predict and you have dynamic enough systems that are connected, you end up in being adaptive. So you can adapt and maybe even prevent things from happening in the first place through that process. So I think that that’s where we currently are. And there is a lot of exciting new things in the future, but I suspect that that’s going to be a separate section of our conversation
10:38 – 10:41
Fabrizio Maniglio: where we talk a little bit more about what’s coming next.
10:41 – 11:24
Yan Kugel: Right. So I think AI has been used a lot, especially in research, right? Because in research, there are not a lot of, you know, red tapes, right? So it’s been used already to detect molecules that can be used as pharmaceutical entities, and it’s much easier to do, but how to use it in the pharma, it is a bit more complex because there are a lot of red tapes and the question of what you can actually do. So for me, when I was thinking about, you know, change GPT and everything that you can do, there are so
11:24 – 12:13
Yan Kugel: many application from what I can just think about as assistance in analyzing deviations and helping with risk management and risk analysis and root cause analysis, as even if it doesn’t do it for you, which is amazingly it can if you give it the data, it can also serve as a guide or a consultant that sits with you and just guides you through the process. And I think that can be quite a breakthrough there. And I see solutions in the business sector and marketing sector that are like mushrooms popping but in pharma we still don’t see much
12:13 – 12:22
Yan Kugel: of it so Do you think this is something that is viable, that it can happen? And will it be happening soon that you will see AI assistants?
12:23 – 13:05
Fabrizio Maniglio: So, yes and no. I think I’ll take it back to your first question of AI and the application of AI. And I would caution to put all the terms in 1 basket because AI is not equal to AI. And I think your question ended up in Gen AI, which is the chatbots and the generative artificial intelligence. But the reality is artificial intelligence is a much wider field than just generative artificial intelligence. And there are quite a lot of applications that are already being used even further downstream of the manufacturing process in the pharmaceutical world, for example.
13:05 – 13:43
Fabrizio Maniglio: So if you think of categorization of things, if you think of looking for similarities in a data set, all of those things are already there and are already being used within the GXP regulated space because it allows to augment human decision-making. So that’s where I would draw, and those are not necessarily generative artificial intelligence, they would fall more into the category of machine learning where the algorithm is still trained but it’s not generating new information, it’s just helping you to categorize or helping to determine if something’s similar. But those are all use cases that augment human
13:43 – 14:18
Fabrizio Maniglio: decision making and I think that that’s 1 of the solutions when we start thinking about how to deploy this in a regulated spaces, well, first look for low-stake environments. Low-stake environments where you have things that are always going to have a human in the loop in this context. And the human in the loop meaning someone I can check, because for creative tasks, coming back to chat GPT or just generally generative intelligence, that can be very helpful to help you brainstorm or to help you get started. And then you’re still the final reviewer that makes sure that
14:18 – 14:56
Fabrizio Maniglio: it actually makes sense and there is still this human in the loop. And the other consideration is always going to be, yes, they are not, artificial intelligence is not perfect, maybe not perfect yet, But I would also always advise to make sure that you know what you compare it with, right? Because neither are we. We are not perfect. Humans make mistakes. And a lot of times when we’re trying to replace a human task with a system, we’re aiming for perfection. And we’re expecting that it’s 100% accurate. But oftentimes, if we compare it to, let’s say, the
14:56 – 15:16
Fabrizio Maniglio: worst possible employee that you may have and his performance, then if it’s better, then on average, that may be better for you in this specific task, or maybe not. But I think those are nuanced distinctions that need to happen when we’re talking about is it viable in the GXP environment.
15:18 – 15:58
Yan Kugel: Right. So I think there are a lot of consideration there. So first of all, we have the validation. So I think that taking something like generic, like a GPT, for example, like that it outputs data. And in terms of validation, you cannot actually validate it because each time the data you will get will be different, right? So you will always need somebody to check it because you can see when you play with JGPT and you ask the same question you will get each time a bit a different result so that’s why it probably cannot be completely
15:58 – 16:14
Yan Kugel: validated to a complete GMP use because they also have the problem of hallucination effect that they just, if they don’t know the answer, they just invent something as if it’s true, right?
16:15 – 16:56
Fabrizio Maniglio: Sometimes, yes. But I would also say that the example you made with validation is always the question. So can you validate it? Well, you cannot validate that it reproducibly creates the exact same response time over time. But that may not be the requirement. So it’s always the question, what are we validating for and what’s the intended use? But the wider question, this is a topic that I started asking myself, is validation even the right tool set to look at this? Because Again, I often say that almost counterintuitively, chat GPT or large language models in general may
16:56 – 17:29
Fabrizio Maniglio: be the most human technology that we have introduced in a while. Not in a sense that it is aiming to replace humans, but it is human in a way that allows for natural language, allows for variability in responses, which we see if we ask 3 employees the same thing, we’ll get slightly different responses that are grounded in the training that they did. So I started thinking about this concept of maybe validation may not be the right way of thinking about it, but treating it almost like an employee. So how would you go about treating an employee
17:29 – 18:02
Fabrizio Maniglio: if you have a position to fill? You would go and look in the marketplace and would make sure that the CVs and the certifications that the candidate brings with him are meeting the requirements that you have for the job. So you can do the same with AI. You can say, okay, does this model meet my requirements? Is it secure enough? Where is the data stored? You do your due diligence. And then when you decide to hire an employee, then you go for onboarding. So that could be the grounding and the fine tuning of a model. And
18:02 – 18:33
Fabrizio Maniglio: so you teach it almost like, okay, I know that you can roughly do critical thinking and you can do this job, and now I’m going to train you how we in our organization do this job. So you go through a training exercise, And then from there, you still don’t expect the employee to be perfect, but you put downstream controls in place to catch any mistakes. And I would treat generative artificial intelligence much akin an employee to say put downstream humans in the loop to make sure that if errors were to happen, you still catch them. And
18:33 – 18:45
Fabrizio Maniglio: ideally, the system is still giving you more accurate outputs than a human would be. So the reviewers will have to catch less and less mistakes down the line. Right.
18:45 – 19:31
Yan Kugel: So that’s an interesting point to look at it. And it makes also quite a lot of sense. So I think when you’re saying, okay, we need another evaluation tool and not per se, validated. So I think probably the risk assessment would be the right, a better tool to assess this. And I think this is where also the FDA is heading because it steps back from, you know, this, you know, way of validation as it was before, and it wants to move more into risk management validation type, where it’s more you assess the risks and you need
19:31 – 20:00
Yan Kugel: to do just a huge report on something that you didn’t evaluate correctly, or you’re just sometimes testing for things that are irrelevant, just to add some pages to the report, right? So it all depends on the user requirements, what you want to achieve and what it can do and what are the mistakes or the issues and how we can catch them and what is the impact of such mistakes on our manufacturing,
20:01 – 20:39
Fabrizio Maniglio: right? Yeah, that’s very crucial and what you’re mentioning. So I think you were hinting at computer system assurance rather than validation, right? Yes. But you raise an interesting point that you should always have a risk assessment and understanding of what’s the intendant’s purpose and what’s the risk of it being used for this. Because there is a huge difference, for example, to use artificial intelligence to generate a draft audit plan, for example, so that the auditor doesn’t have to start from scratch and then he still takes that audit plan and refines it and then goes and performs
20:39 – 21:08
Fabrizio Maniglio: the audit. That’s a very low risk implication or application of an artificial intelligence because even if the things are not 100% accurate, even if, to your point, it may hallucinate a little bit, there’s still a human that takes that as a starting point and then makes sure that it makes sense for the audit that the auditor is going to go perform. So that’s a completely different use case to saying, Oh, I’m going to deploy an artificial intelligence that will release my batches for me without any oversight. So that’s a completely different realm and a completely different
21:08 – 21:23
Fabrizio Maniglio: piece that will require a whole lot of oversight in a different way and a lot of assurances to make sure that you can actually trust it. And I think we’re a little bit further away from the second point, but the first point is very feasible already now, I believe.
21:25 – 22:07
Yan Kugel: Yeah, so I agree with you completely on that. And you mentioned before that the issue that a lot of companies are facing, or not the issue, but something that I also feel that sets everybody in the manufacturing and the approvers back is the use of multiple systems which are not connected to each other. So I’ve seen it as well during my work where of course you have the lean system, sometimes you have the QMS, sometimes you do validation of systems in a completely other software, sometimes the SOPs are also completely not connected. It can be also
22:07 – 22:54
Yan Kugel: the deviation and which are not connected also to the QMS or the batch release, etc, etc, etc. Right. And you mentioned that the future that they, or the thing that is the easiest to solve might be connecting everything together, right? And how do you see this happening? I’m sure that also, so I know that we said that we will not speak on behalf of Honeywell, but you probably, when you work for Honeywell, there are user requirements that you see, and you probably tackle those problems a lot that companies want to connect their limbs, let’s say to
22:54 – 23:11
Yan Kugel: Honeywell and But then the limb system should have open API or different tools that it allows you and gives you the permission to connect to it. So how do you see this issue being solved or tackled or what are the issues that are there in this regard?
23:11 – 23:58
Fabrizio Maniglio: I would probably start by saying most modern systems have in 1 way, shape or form, the ability to have APIs connect outwards to other systems. So I don’t see that necessarily as a limiting factor. Of course, there is also still a surprising amount of homegrown systems that were built in-house. The situation there may be slightly different. I don’t think it’s necessarily a technology challenge of saying it’s hard to connect 1 system to the other. That may be straightforward. We have been doing integrations for a long time. I think that the idea of data lakes, for example,
23:58 – 24:30
Fabrizio Maniglio: exists also for a very long time. And putting all the data in 1 place so that you can then start figuring out what to do. I think where we’ve started to kind of change a little bit the way that we’re thinking about that is oftentimes having all the data in 1 place just ends up having a data lake turn into a data swamp, so to speak, and then you don’t really know what to do with the data. So oftentimes it’s better not to think about the how of connecting systems, but start with the outcome in mind.
24:30 – 24:59
Fabrizio Maniglio: So I would always recommend instead of asking, can we connect 2 systems, to ask the question, what are we trying to achieve? What is the outcome we’re looking for? And maybe in your case, it’s connecting LIMS with a QMS. And in that case, you are expected, the connection is just a means to get the data connected in a way, to get you a certain outcome. So I would always start with the outcome in mind and say, okay, what are the outcomes we want to deliver? And then how we get there, that’s the thing that is to
24:59 – 25:18
Fabrizio Maniglio: be figured out. And often what we are starting to do more and more is going to the realm of having data pulled from systems at the time that it needs to be so that you have the right data in front of the right people at the right time in the right format so that the decisions can be taken.
25:21 – 25:59
Yan Kugel: Right. So I think that’s a very important point, data swamp, right? So you get a lot of the information, but the question is Who looks for it and what is the purpose and why? So I think that having a lot of data and structuring it and understanding what can help you. I think that a lot of companies don’t work with quality dashboards, for example. They have no idea, even quality heads in some companies, I think have no idea how many deviations, how many repeating deviations they have per year. If you ask them that, they’ll probably, oh,
25:59 – 26:39
Yan Kugel: you know what, I need to go to some Excel, start counting. And the same for change control. It’s not always being monitored or issues with suppliers. So I think a lot of those things can be easily solved with dashboards. But also if you’re talking about AI, right? So if you teach AI to sort through the right data for you, let’s say even predictive AI that can say, you know what we are seeing, we are catching some anomalies that we, it predicts that it will result in a deviation in 5 batches, you will have a malfunction in
26:39 – 26:52
Yan Kugel: the system or something like this. So I know that some companies already like working in this, especially in maintenance and tools, but what is your perspective in general and quality in the different direction it can be used?
26:56 – 27:08
Fabrizio Maniglio: So if I reframe the question, you’re asking about predictive capabilities, right? So you make the case of predictive maintenance. I think that’s something that…
27:08 – 27:28
Yan Kugel: Right. So different uses of… We have the data. So what different uses we can do? So predictive can be 1 thing that I’m thinking about, like doing dashboards. But I think the most interesting thing is actually because our topic is more AI. So I think that it would be interesting to touch upon.
27:28 – 28:04
Fabrizio Maniglio: Yes, absolutely. I think prediction, I mean, prediction doesn’t necessarily require AI. And I had this conversation already a couple of times where the objection basically is, we have been doing predictions for a long time. You can do multivariate analysis, you can look at data, it’s basically statistics, and then you draw your prediction. And that’s true, prediction requires data. And also, to your point, I think we require data to be connected in certain ways, back to the framework of you need to have the ability to understand why things are happening and why things are connected.
28:05 – 28:26
Yan Kugel: Right. But is it a manual process? Like you were saying, okay, we can do it. It’s statistics. But the question is, is it so far a manual process Or are there already systems which you’re saying there is a statistic strands in the background and then there is a red light that lights up and something is not right?
28:27 – 29:06
Fabrizio Maniglio: I think it has been more manual than it needs to be now. And it’s definitely become easier with using artificial intelligence to solve certain of those problems. I think the interesting part with statistics or predictions with AI comes not necessarily from the prediction, which again you could probably do already, though it may have been more manual and cumbersome, with AI that becomes more easy. I think the new concept that has come up is now emergence of insights. So with enough information, a model can surface insights that you didn’t think of. So the problem with normal predictions
29:06 – 29:36
Fabrizio Maniglio: would have been that you need to know what you’re predicting for. So you need to understand, okay, I want to know when this is going to fail, and I know all these data points tell me when it’s going to fail, and that will help me to predict based on the data points where I’m going. The interesting part with emergencies is that you will have new insights or things that you didn’t know that are now shown to you as potential things that you want to take into account. I think that becomes very interesting when we go into
29:36 – 30:12
Fabrizio Maniglio: development. The other thing that is happening is almost on this curve where initially we have like normal artificial intelligence with machine learning. So we talked about categorizing things or finding similar things. But then from there, we move on to GenAI, which we are talking about now, which is able to generate. Then we now talked about prediction, which is the next step of, okay, we can predict things start to emerge. And the next point after prediction, I think, is going to be the ability to have potentially autonomous agents, autonomous systems that can do many more things together.
30:12 – 30:51
Fabrizio Maniglio: And the jump from the 1 to the other is the notion that not only can things emerge, but you can now train models or start being predictive with things that can be taught. So the notion there, or the difference, is with systems that you can say, okay, I can train the system to react to situations I’m already aware of. But with a person or an employee, you train them in the rules of the game, and then that person will be likely able to manage any situation, even if it’s a new situation. And that’s going to come
30:51 – 31:02
Fabrizio Maniglio: when things and systems can be taught and when systems based on data can start implicitly learn what the rules are. I think those are 2 quite game-changing things.
31:05 – 31:50
Yan Kugel: Right, so I agree with you on that. So that will be quite interesting to see emerging in pharma indeed. And you mentioned it yourself, right, that the pharma is usually, especially maybe not the pharma as a whole, because I think the commercial sector is very digitalized. So I think it’s more the manufacturing part that is always lagging behind. And it’s clear, it’s especially because the issue with the validation and the regulations and the difficulty to implement new system. So do you think this is 1 of the reasons why a lot of innovative companies stay away from
31:50 – 31:59
Yan Kugel: pharma, do you see it changing where more companies start bringing those solutions to pharma and taking the risks?
32:02 – 32:38
Fabrizio Maniglio: I hope so. I’m a hopeless idealist at times, So I hope that that’s where it was going. I suspect that the pharma industry may be ripe for what I tend to refer to as almost like a Kodak moment of if you don’t hop on board and if you don’t figure these out, then you’ll become obsolete in time. And I believe that because now is the time to understand and figure out how to use these systems. I also believe that there will be a point of no return where no matter how much you try starting from a
32:38 – 33:18
Fabrizio Maniglio: certain point in time, you will never be able to catch up with companies that have already figured out how to use these tools. I also hope that the industry as a whole realizes that it’s up to us to actually drive this change and oftentimes what I see in our industry is a reluctance to move and to wait for regulators to figure these things out. Now, sometimes that may work, but regulators and regulations and legislature doesn’t move that quickly. It’s often a cumbersome and slow process, whereas the technology that we are talking about now moves very, very
33:18 – 33:51
Fabrizio Maniglio: quickly. So I think it’s unrealistic for us to expect that we will have a regulatory framework that we can follow blindly to say, oh, just do this and this and this, and then using AI is going to be fine, or use these systems and you’re going to be fine. It’s for us to justify what is okay and what is not, in what use cases. And there, I think, there is huge potential. The other thing that I kind of always want to mention is The reason why I’m so excited and passionate about certain artificial intelligence models that
33:51 – 34:26
Fabrizio Maniglio: do certain things is, oh, it generates a picture, it generates a video, it can generate music. The reason why all of the industry should pay attention to that is because it’s not what it does. It’s the technology that is behind it that is interesting to understand. Because we’re seeing all of these models pop up where data is readily available for developers to use to train their models. And Just imagine the same capabilities applied to a data set that is specific to the life sciences. Maybe you apply it to your distribution network and then it learns how
34:26 – 34:36
Fabrizio Maniglio: your distribution works and is able to simulate that. Those are very interesting use cases that I see the future holding for us if we actually embark on this journey together.
34:38 – 35:22
Yan Kugel: And do you think those kinds of ideas should usually come from the companies themselves that are different entrepreneurs that see the need in it and then try it out? Or should it come from certain companies that we know that big companies, as you mentioned, they build the systems around their manufacturing. And from 1 side, it’s making it harder right now to switch, but on the other hand, they were the first ones to digitize. So what do you think, Should the push come from the industry where companies should think, okay, what we need and then try to
35:23 – 35:37
Yan Kugel: find companies, partner with them and just build the tools? Or should more entrepreneurs who serve the pharma be more open-minded and try out different solutions?
35:40 – 36:20
Fabrizio Maniglio: So, I think that there’s multiple aspects to your question that I would like to address. On 1 side, I think that trying to understand the technologies and playing around with it and applying it, again, in low-stakes scenarios within your organization is probably a good thing to do now on your own without looking outside for finding people to do things with initially, because that helps you to understand what the technology is capable of. Now, at the same time, I’m not advertising to just become an IT company, because usually all the companies in the lab science have a
36:20 – 36:57
Fabrizio Maniglio: very clear mission around bettering patient outcomes and patient safety and they should be focusing on that. But the reason why education and understanding of capabilities is so important because that’s where innovation comes from, is understanding what is possible and being able to apply it to your world. So if you know, for example, that a system with enough training data is able to implicitly learn how things work, then maybe you say, okay, what if I were to apply this to my manufacturing data? And with that, you could then say, okay, if I apply this to my manufacturing
36:57 – 37:18
Fabrizio Maniglio: data, then I would be able to simulate and predict what happens with my manufacturing data. I will be able to ask questions and that will help me in this way. So it’s up to the industry and the individuals to come up with use cases. And then it’s up to partners and software companies to then help develop those solutions together.
37:20 – 37:51
Yan Kugel: So do you see a lot of enthusiasm among your peers and colleagues who are either directors of technology or systems in the pharma, or your peers who are also business developer directors at various service providers? So do you see the rush and the excitement as well in other people in the industry?
37:52 – 38:28
Fabrizio Maniglio: I mean, you tend to natively surround yourself with people that have a similar predisposition. So I guess the easy answer is yes, there is a lot of enthusiasm. I think there is a lot of hype around artificial intelligence in general coming top down from management, because it has been quite visible. And there is a lot of pressure and push to implement artificial intelligence around a variety of different organizations. Now, that can be good because it can fuel innovation. It can also be dangerous because, as I always try to advertise, artificial intelligence is just yet another tool
38:28 – 38:58
Fabrizio Maniglio: that we can use to solve problems and deliver outcomes. So it shouldn’t be mistaken with a silver bullet that does everything for us and it shouldn’t be just… So you shouldn’t do AI for the sake of doing AI. You should have, again, back to having an outcome in mind. I think it’s important to have an outcome in mind and say trying to achieve this and then maybe AI is 1 part of that solution. Maybe it’s the whole solution but maybe there is other ways of achieving that that don’t require AI. But I think there is a
38:58 – 39:07
Fabrizio Maniglio: lot of excitement about the possibilities and the art of the possible of where we can go to if we are leveraging those technologies efficiently.
39:09 – 39:52
Yan Kugel: And you mentioned that you surround yourself with the right people who are visionary and they are hopefully also dreamers as well because they want more for the industry. So how do you keep up with the trends? What are your sources to keep up with the trends if somebody also wants in the industry to pick up on this AI trend and see what types of AIs are there, because we all hear about generative language models, but there are so much more, as you said, So what do you like to… Are there conferences, blogs? Is this something you
39:52 – 39:53
Yan Kugel: can share?
39:55 – 40:37
Fabrizio Maniglio: So in a shameless self-promotion, just follow me and potentially I can help you with keeping up with the news. But of course, if you want to do it on your own, what I do a lot is I have a few newsletters I subscribe to that I trust. I go to Twitter or X for some information. I also have a little news aggregation tool that helps me to kind of sort through and sift through information and find information out. There is also a lot of free tools if you just want to learn the basics of artificial intelligence
40:37 – 41:14
Fabrizio Maniglio: machine learning or just generally data science. There’s quite a lot of free tools out there that prominent companies have put out there. There’s some free courses by Google, for example, or Microsoft that just offer to give you an idea of what this is all about. There’s plenty of courses on prompt engineering if you want to figure that out. And then I also get a lot of inspiration and information from, again, the group of colleagues that I’ve surrounded myself with in and around LinkedIn. That’s also a good source of information at times where you find quite a
41:14 – 41:44
Fabrizio Maniglio: lot of good articles. Also always with kind of the viewpoint of this is somewhat how relevant to business and not just fun. So those are a few things that I do. And I’m happy to answer any specific questions. If someone wants to reach out to me directly, it’s like, how do you solve this? I’m happy to share my experience. But the reality is also, if I’m completely honest, it’s a little bit of a struggle still, because there is so much information. I usually don’t have enough time to read everything that I’m interested in. I need to
41:44 – 41:49
Fabrizio Maniglio: triage a little bit for myself and pace myself. But there is lots of interesting information out there.
41:52 – 42:33
Yan Kugel: Great, thank you for this advice as well. So, just another question about the future of use of AI. So we said there are so many possibilities, but many of them depend on the regulator as well. So if you could draw the next stages of 3 years, 5 of maybe 10, What do you think will happen in the industry throughout those years? And what will be the quickest to establish? And what is like, it’s still maybe sci-fi, but we probably will get there quite soon.
42:34 – 43:10
Fabrizio Maniglio: Okay, so here is what I would probably… I would probably change your timelines ever so slightly, because I don’t think I can… I don’t feel confident making a prediction for 10 years, because of the speed of how technology is moving at the moment. I think that a lot of predictions that go that far out will likely be wrong because we don’t, we cannot understand and it’s not trivial to figure out what, how these systems will amplify themselves and each other to create much quicker results, much quicker than we thought. So there is a graph that projects
43:10 – 44:00
Fabrizio Maniglio: where general artificial intelligence, for example, would hit. And currently we’re somewhere around 2027, where we potentially would have an artificial intelligence that’s considered general artificial intelligence that would be doing all the things, roughly speaking, probably better than untrained humans would in any field. And that then itself would then amplify again. But I think right now, what we can already do is use this notion of augmenting human productivity, effectiveness, human decision making, and leverage artificial intelligence and other cutting edge tools to help us do things better that we have already been doing. So that could be helping
44:00 – 44:40
Fabrizio Maniglio: you to summarize, helping you to categorize, helping you to write things faster, brainstorm things, help you with root cause analysis. All those things are already possible now. I think in the future, in say the next 1 to 2 years, I think what we’ll see is we’ll see the technology deployed to solve problems that maybe were not previously solvable. So going into the direction of, hey, for example, unstructured data is always a topic that comes up in that realm, where you would then go and say, how can I take all these stacks of paper or all these
44:40 – 45:13
Fabrizio Maniglio: unstructured documents and do something with it? And I think that that’s something that we’ve seen technology being able to handle. I think that in 1 or 2 years we will start seeing solutions even in the life sciences that will leverage that to do much more with data. And with that we will be able to train better models to deliver even better outcomes. And from there I suspect at some point in time, we’ll get to an interface into our data that is completely different from how we interface with data now, which will be very conversational. So I’m
45:13 – 45:43
Fabrizio Maniglio: almost envisioning a general chat agent or an AI that you can talk to, to virtually do anything that you’re doing today just by having a conversation saying, I think we should be doing this or I’m wondering what the situation looks like here and here in this context and you would get the information and from there you can drill down and you can have a conversation and figure out what. And always have the guidance. I think you brought that up. So, almost like an agent that can guide you and help you and support you, but also that
45:43 – 45:53
Fabrizio Maniglio: you can interface with the data with and talk about anything that you want. I will probably put that at 5 years to be brave and dreamy that we will have something along those lines.
45:54 – 46:46
Yan Kugel: Yeah, so I agree with you about that. So a while back, I listened to a podcast with a futurist and he said that people are quite modest in the way technology progresses because it is quite logarithmic. And if we are looking at computers like 20 years ago, we are doing like trillion more computations per second, which is like incredible. And the way we can progress, we allow ourself is astronomically higher than we think. And I think it connects with it as well, is only to use it and not to be left behind and behind the industry.
46:47 – 47:01
Yan Kugel: When we’re talking about pharma manufacturing quality and so on and try to be on pace and hopefully the regulators can start helping with that as well and sort of hindering and hopefully we, you know, slowly see that happening.
47:04 – 47:41
Fabrizio Maniglio: And I also think just to add to that, we often think, and I think the notion in the industry is that regulators are reluctant at best to implement or to support these. But oftentimes when I’m having conversations with regulators or seeing what they’re doing within their own 4 walls, then they’re already playing with all these different things. They’re hiring data scientists, training data scientists, deploying artificial intelligence models in-house to analyze data. And they’re figuring things out for themselves. Now, they may not be willing to say, hey, here’s a carte blanche. This is how you need to
47:41 – 48:15
Fabrizio Maniglio: do your AI. But they’re definitely already getting ready to be able to understand and inspect it. Getting ready to use it in-house for their advantage. And I think very open to go there. There’s even the saying that it’s almost like a moral imperative for life sciences companies to actually use cutting edge technology to better outcomes for patients. So we shouldn’t be just waiting for regulators to say, this is okay. I think they’re on board with this is not going to go away and it’s going to be part of our future. And we just need to figure
48:15 – 48:16
Fabrizio Maniglio: out how to get it.
48:18 – 48:34
Yan Kugel: Great, great Fabrizio. So very fascinating talk. So before we wrap it up, is it something that we didn’t touch and you feel it’s important to share about the digitalization AI that we left out? I
48:34 – 48:45
Fabrizio Maniglio: mean, we can probably talk for another 2 or 3 hours without exhausting all the different avenues and topics we can touch on. But I think that this was a great first start and a Great first conversation.
48:46 – 49:21
Yan Kugel: Great. Thank you, Fabrizio. So to the audience, everybody who’s listening, make sure to follow Fabrizio Manilio on LinkedIn. He shares fascinating information about AI, and also quite very interesting images that I generated to follow his posts. Very interesting, fascinating. And of course, probably we’ll see him on more of our webinars, so stay tuned on that hopefully. And on that note, Fabricio, thank you very much for joining me today and have a great day ahead.
49:21 – 49:24
Fabrizio Maniglio: Thank you very much for having me and thanks everyone for listening in.
Tagged as: AI, Digitalization.
Our guest Harsh Thakkar shares valuable insights on building leverage, creating content, and the importance of online presence in the business world.
Copyright © 2023 Qualistery